Researchers Developed A Sophisticated AI Algorithm That Can Identify Therapeutic Targets For Both Glioblastoma Multiforme (GBM) And Other Cancers
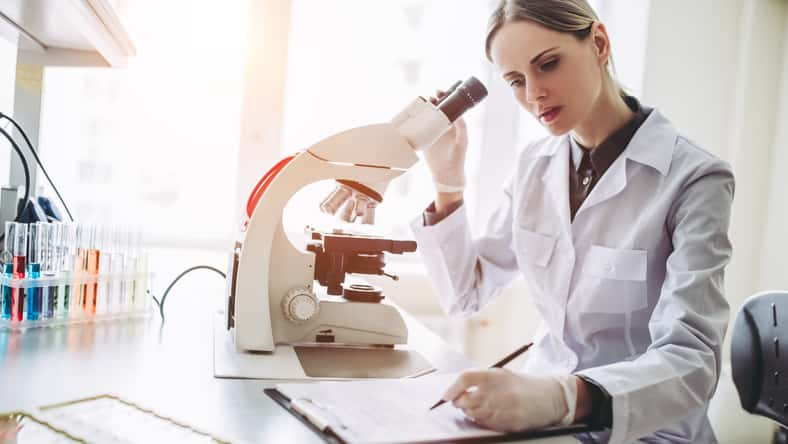
Researchers from the University of Miami Miller School of Medicine recently developed a new AI algorithm that can perform advanced computational analysis. In doing so, possible therapeutic targets for glioblastoma multiforme (GBM), as well as other forms of cancer, can be identified.
This finding could have significant impacts on the future of GBM treatment– since GBM is a fast-growing, aggressive, and often fatal brain cancer.
Additionally, the researcher’s work may pave the way for advanced treatments of certain lung, breast, and pediatric cancers.
“Our work represents translational science that offers immediate opportunities to change the way glioblastoma patients are routinely managed in the clinic,” said Antonio Iavarone, the study’s senior author.
“Our algorithm offers applications to precision cancer medicine, giving oncologists a new tool to battle this deadly disease and other cancers as well.”
The AI algorithm is called Substrate Phosphosite-based Inference for Network of Kinases– otherwise known as SPHINKS.
SPHINKS actually used deep-machine learning to enable the research team to identify two protein kinases known as PKCδ and DNAPKcs. Then, the AI algorithm helped the scientists experimentally validate the protein kinases’ association with tumor progression among two GBM subtypes.
In other words, this validation enabled the team to identify PKCδ and DNAPKcs as viable targets for potential cancer therapies.
Protein kinases are already currently used as vital targets in precision cancer medicine in order to tailor a patient’s cancer treatment to their specific needs. Clinicians target drugs toward the most active kinases– which the study’s authors refer to as “master kinases.”
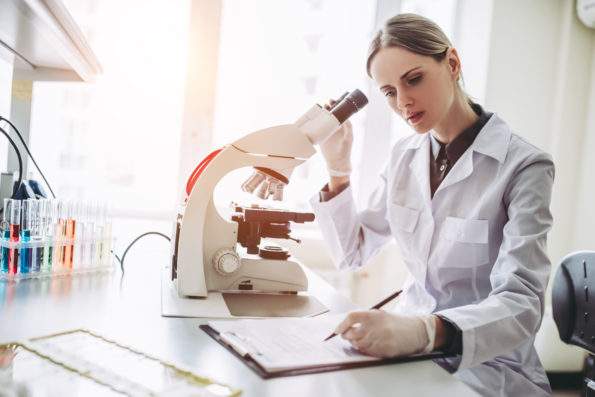
Vasyl – stock.adobe.com – illustrative purposes only, not the actual person
Aside from identifying these master kinases, though, the researchers also went one step further. By using “patient-derived tumor avatars”– or tumor organoids from patient samples that were grown in the laboratory– the team found that targeted drugs that interfere with master kinase activity can prevent tumor growth.
In the past, Dr. Iavarone and his team also reported a new classification of glioblastomas. This was completed by identifying critical tumor cell traits, as well as grouping GBM patients together based on the likelihood of survival and their tumor’s drug vulnerability.
So, during this new study, the researchers independently confirmed the classifications via numerous omics platforms– including proteomics (proteins), genomics (genes), lipidomics (fat), metabolomics (metabolites), and more.
SPHINKS is also able to leverage machine learning and refine these datasets, creating an interactome– or a full biological interaction data set.
Through this interactome, kinases that prompt abnormal growth and treatment resistance in every glioblastoma subtype can be pinpointed.
So, multi-omics data can essentially generate novel algorithms that are able to predict what targeted therapies would provide optimal therapeutic options depending on a patient’s glioblastoma subtype.
“We can now stratify glioblastoma patients based on biological features that are common between different omics. Reading the genome alone has not been enough. We have needed more comprehensive data to identify tumor vulnerabilities,” explained Dr. Lavarone.
This breakthrough is monumental– since the five-year survival rate for glioblastoma patients is below 10%.
And while various new drugs are currently being developed for potential therapies, being able to identify the molecular mechanisms that drive each patient’s disease will help improve treatment offerings.
According to the researchers, the SPHINKS algorithm is capable of just that and can be readily integrated into molecular pathology labs. Afterward, the team believes this approach could benefit up to 75% of glioblastoma patients.
And despite SPHINKS being first tested on glioblastoma, the AI algorithm is also applicable to various other cancers. More specifically, the team found the same kinases that drive cancer in lung, breast, and pediatric brain tumors.
So, the researchers believe this study could be a launching pad for a brand new clinical trial.
“We are exploring the concept of basket trials which would include patients with the same biological subtype but not necessarily the same cancer types,” Dr. Iavarone detailed.
So, if patients with glioblastoma or lung or breast cancer have molecular features that are similar, they may be included in the same trial– as opposed to conducting various trials.
Dr. Iavarone believes this could potentially bring effective drugs to a greater amount of patients much faster.
To read the study’s complete findings, which have since been published in Nature Cancer, visit the link here.
If true crime defines your free time, this is for you: join Chip Chick’s True Crime Tribe
Sign up for Chip Chick’s newsletter and get stories like this delivered to your inbox.
More About:Science